The Next Great Tech Revolution
AI research at Rice is fueling significant breakthroughs, plus debate about the public good.
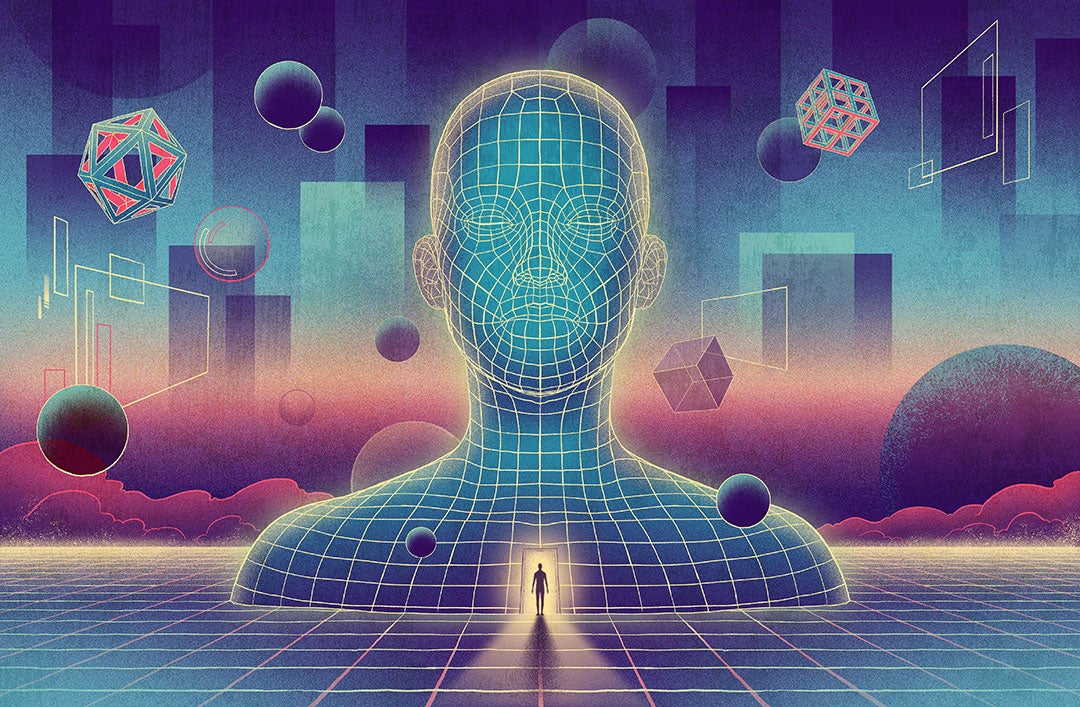
Spring 2024
By Hilary C. Ritz
Illustrations by Antoine Doré
Angela Wilkins, until recently the executive director of Rice’s Ken Kennedy Institute, is definitely pro-artificial intelligence. Even over our Zoom call, her enthusiasm about it is uplifting, and it’s entirely with that kind of positive energy — not distrust or anxiety — that she says AI has “seeped into everything.”
“It’s in every field now. Everybody’s doing it,” she adds. “They’re either making AI that works for them, using AI that works for them, or they have a collaborator that’s doing it for them.”
When I ask her why AI is so important, she says, as if it were perfectly obvious, “It’s important because science is important. AI allows us to do better science.”
For Wilkins, who is newly appointed to the Texas AI Advisory Council, AI is simply the next great technological revolution, following the printing press, the personal computer and the internet. “Now we can see something that might help make the world a better place,” she says. “Hopefully fix climate change and hopefully make the world more equitable. I’ve never seen anything quite like what’s going on now in the context of, just, people see what the possibilities are.”
But Wilkins’ enthusiasm isn’t blind faith, nor blind optimism. “We need explainability, we need responsibility. We need to make sure that it’s fair, it’s equitable. For technology to influence the way we do things, it has to be done right,” she says. “This is something we really, really care about.”
All this is really prelude to the conversation I set out to have with Wilkins, which was to understand the full breadth and depth of AI research on campus at Rice. As it turns out, this is a challenging task. “We have people who do everything,” Wilkins says. “As a whole, we’re covering the entire field.”
I decide to focus on several key projects from disparate areas in order to showcase the full range of research at Rice. When I ask Wilkins to suggest projects, she exclaims, “It’s so hard to choose! I love everybody!” But she admits that she’s particularly excited about the collaboration between Rice and Houston Methodist, where robots will be used to train nurses, and soon I’m off to the brand-new Ralph S. O’Connor Building for Engineering and Science to learn more.
Embodied AI for better health care
At the O’Connor Building, computer scientists Lydia Kavraki and Vaibhav Unhelkar and grad students Pam Qian, Qingxi Meng and Carlos Quintero Peña meet me in their fourth-floor lab. There, Kavraki tells me, “The health care field lost one-third of its workforce since COVID-19, so this means that there is a shortage, and they don’t have the instructors to train the nurses.” According to their partners at Houston Methodist — Shannan Hamlin, Nicole Fontenot and Hsin-Mei Chen — it’s a real problem.
Enter a robot that looks less like what I imagined when I thought “robot” and more like a Roomba with a tall camera stand on top of it. But I soon understand that the minimalism is by design, making the robots unobtrusive, maneuverable and cost effective. Their first application will be to help nurse trainees learn how to maintain a sterile field while applying dressings to wounds. Qian and Meng demonstrate how the system tracks hand and body movements and flashes a warning when trainees break rules, such as reaching across the sterile field.
Many of the techniques that have been developed in AI really get their final tests in the embodied AI systems.
Many milestones are yet to come, like programming the robot to continuously position itself to get the best view of the trainee’s movements while staying safely out of the way. “There are a lot of systems that have to come into play,” Unhelkar says, listing areas of research such as “imitation learning” and “perception-aware motion planning.”
Kavraki adds, “Many of the techniques that have been developed in AI really get their final tests in the embodied AI systems, because there you cannot afford to make mistakes. You cannot afford to have this robot collide, even if it is 1% of the time.”
As far as the team knows, this is a unique application of embodied AI. And sterile field training is critically important, considering that, according to the CDC, one in 31 U.S. patients acquires an infection in association with their health care every day. But this is just the beginning of what these robots will be able to do.
This is an exciting project, and I soon discover that the potential impacts of my next research subject could be equally significant.
Predicting floods faster
The focal point of Arlei da Silva’s small, tidy office in Duncan Hall is a huge white board filled with formulas and diagrams that I find incomprehensible. Luckily, the softspoken professor of electrical and computer engineering is skilled at explaining complex ideas, even if he’s so quiet I’m worried that my voice recorder won’t pick him up.
Da Silva is working on multiple grant-funded projects that will make cities of the future more resilient in the face of dangers like floods or cyberattacks. One key project aims to improve flood prediction in complex urban environments, with Houston as the major case study.
“The current approaches for flood prediction are based on mathematical models that try to capture approximately the physics of the problem,” da Silva explains. “But the problem is that these approaches are very slow.” Using AI and machine learning, da Silva and his graduate student Arnold Kazadi have shown in some of their recent results that these calculations can be done “a hundred times faster than some of the best existing solutions.”
Instead of, perhaps, an hour, a flood prediction model can now be run in seconds, and while that raw difference might not sound too impressive out of context, “if you’re thinking about evacuation, it makes a huge difference,” da Silva says. “The water goes up so fast, that might say who can evacuate and who can’t. Also, if you’re trying to account for multiple possible outcomes, if it takes a few seconds versus one hour, you can look at a hundred more outcomes.
“And if you know how bad flooding will be in certain areas, now you can combine that with demographic data to see how many people will be impacted, and then you know how many firemen should go there to help these people evacuate, or to rescue them.”
The best part is that these new lightning-fast calculations are very close in accuracy to the ones made by classical methods, at least when looking up to a few hours ahead, da Silva says. His team is working to improve the accuracy even more and to extend how far into the future the predictions extend.
He says they have a lot of “confidence” in how the project will go, then humbly corrects himself to “hope” — but perhaps that’s just the kind of quiet confidence that doesn’t need to shout to be heard.
Democratizing AI
I soon learn that da Silva isn’t the only humble faculty member on Rice’s campus. Data scientist Christopher Jermaine twice refers to his AI research as “plumbing,” meaning that it’s the unglamorous stuff that happens behind the scenes, but in reality, his current research project could make AI dramatically more accessible to organizations around the world while also diverting millions of older computer processors from landfills.
The problem he’s addressing, Jermaine explains, is that training AI has become cost-prohibitive for many organizations. This task typically uses processors called GPUs (Graphics Processing Units), and to process the sheer volume of data required, an organization might need a cluster of anywhere from eight to a thousand state-of-the-art GPUs that cost as much as $30,000 apiece.
There’s all sorts of used hardware floating around that people are discarding because they want to get these newer, bigger [processors].
The solution is to “break apart the training task and get it to run on a lot of older devices,” Jermaine says. “There’s all sorts of used hardware floating around that people are discarding because they want to get these newer, bigger ones, and they’re actually incredibly capable computationally. You can buy a used but very capable server on eBay for, say, $4,000.” Using his solution, two of those could deliver roughly the same computing power as a new GPU.
I calculate that an organization needing about 100 new GPUs’ worth of computing power could reduce its costs from roughly $3 million to about $640,000. Jermaine believes that these cost savings could help democratize access to top-of-the-line AI models.
So what’s involved in getting AI software to run on these lower-power devices? Jermaine explains that while old processors are capable of making the calculations needed, their memory is too limited. He and his team of grad students, led by Daniel Bourgeois and including Zhimin Ding, Xin Yao, Sleem Abdelghafar and Sarah Yao, have developed a working prototype of software that addresses this limitation. The program automatically analyzes and breaks apart the computation tasks, using a type of advanced math called “Einstein summation notation” to specify the computations, and loads them into memory just as they’re needed, making the best use of the memory available.
Importantly, Jermaine’s “einsummable” method can work for any type of AI training software — and, potentially, any type of high-performance computing. Not bad for plumbing.
Assessing AI assessments
As I'm wrapping up my story, Provost Amy Dittmar gives me another name not to miss: data scientist Fred Oswald. Unlike the other experts I’ve spoken to, Oswald is in the School of Social Sciences and the Jones School of Business, where he teaches industrial/organizational psychology. He has also devoted much of his career to employment testing, going back to when tests were given with paper and pencil, so he has a long-range perspective on the snazzy new AI-powered tests that analyze and score gamified assessments and asynchronous video interviews.
Oswald’s guidance is that the new AI tests, for all their innovations, are not and should not be exempt from the professional standards that have been refined over decades. Tests need to be reliable, valid and fair — the trinity, he calls it — regardless of the methods used.
“We have thought, as employment test developers, long and hard about what makes for a good test,” he says. “The technologists are thinking more about the problem of ‘How do I get these data to this technology to then produce scores through the algorithm?’ Whereas we’re coming at it from the perspective of ‘How do we develop scores that are based on content that is relevant to jobs and relevant to selection?’
“To the extent that algorithms are not transparent, to the extent that data are messy, and, frankly, to the extent these principles are being ignored, we need to say, ‘Hey, listen. We don’t expect tests to be perfect. We do expect tests to be developed responsibly.’”
Oswald is currently working with grad students to assess what kinds of promises vendors are making about these new tests — and what kind of evidence they offer to support those promises. He’s also working on an NSF-funded project, along with principal investigator Julia Stoyanovich at New York University, to discover how HR professionals are actually reviewing, choosing and utilizing these tests — and what kind of pitfalls they’re encountering. “You can imagine a company without knowledge of AI or of tests, even; they just want better, faster, sooner, cheaper,” he says. “How does the company know the vendor is providing a tool with reliability, validity and fairness? Sometimes they don’t even know the right questions to ask.”
The big questions
These kinds of concerns are shared by my final interviewee, Moshe Vardi, albeit at a larger scale. A chaired professor of computational engineering, Vardi has been studying AI at a fundamental level for decades. He asks the big questions, like “What is the nature of intelligence?”
Of late, his research has been in understanding and modeling, through neural networks, the evolutionary split in human intelligence between the so-called lizard brain at the base of the skull, which provides us with quick instinctual responses, and the large forebrain, which allows us to deliberate and reason through logic. “I’m trying to be in the middle,” he says, “trying to build a bridge between fast thinking and slow thinking.”
Vardi is quick to tell me that his own brain is split on the topic of AI, fueling more big questions. “My left brain is doing [all this research]. My right brain is in a panic: What is going to happen? Because people in computing and AI see very, very clearly how the center of gravity has moved to industry. And industry is not going for the most good, it goes for the most profit. So that’s what I lose sleep over. What will it do to humanity? What will it do to the planet?
My left brain is doing [all this research]. My right brain is in a panic: What is going to happen?
“Most people I know, they really would like to see their research used for the public good. I can see that many, many good things will happen. But I’m also very nervous.”
One piece of good news is that the White House has taken an important step in the right direction with its October 2023 executive order on “Safe, Secure and Trustworthy Development and Use of Artificial Intelligence.” As Wilkins says, “One of the things to come out of this executive order is that industry will need to understand the technology and use it the right way. For me, this was a flag in the ground.”
Wilkins’ optimism is infectious. But I find myself compelled to close my own research into AI with Vardi’s final big question: “So how will it work out? I don’t know. It’s an amazing drama.”