New Models for Detecting Skin Cancer
Roxana Daneshjou is working to make skin cancer screening more inclusive — and more accurate.
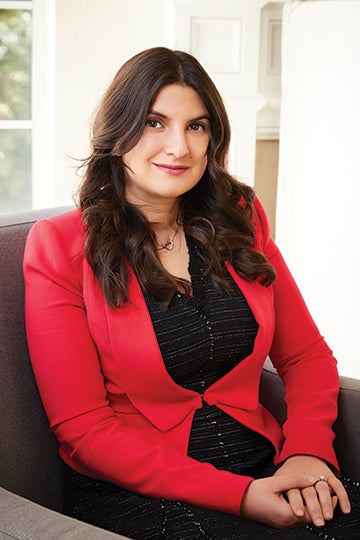
Spring 2023
By Rachel Fairbank
According to the American Academy of Dermatology, 1 in 5 Americans will develop skin cancer during the course of their lifetime. If caught early, these cancers are highly treatable, with an impressive survival rate. However, when it comes to early detection and diagnosis, patients encounter major barriers, including limited access to a dermatologist and physicians who are insufficiently trained about the signs of skin cancer in a diverse set of patients.
“Historically, there is a very large lack of representation,” said Roxana Daneshjou ’09, a clinical researcher in dermatology at Stanford University. “It goes back to the lack of representation in textbooks and educational materials.” Existing images and training are disproportionately focused on what skin cancers look like in white patients, while the warning signs can look very different in non-white patients.
For example, a basal cell carcinoma is typically described as being pink and curly, which is what it looks like on white skin, but it is often pigmented on brown skin. Without proper training for physicians, these carcinomas can go undetected for a longer time. As a result, minority patients are often diagnosed with skin cancer later than their peers and consequently experience worse outcomes in terms of treatment and survival.
When it comes to helping patients access dermatological care, new AI programs can screen images of potentially problematic lesions and make predictions as to which ones need to be referred to a specialist. “These tools could help empower physicians who are not dermatology specialists to help make decisions,” Daneshjou said. Given that an estimated 3 billion people worldwide lack access to a local dermatologist, this type of tool can help patients receive critical referrals.
However, as with the current training methods for detecting skin cancers, the images used for training these AI programs lack diversity. “The exclusion of images of diverse patients from these models is a huge problem that is going to perpetuate existing disparities,” Daneshjou said.
Daneshjou and her collaborators recently published research showing that current AI models were less accurate at detecting cancerous lesions in images of minority patients. However, they also showed that accuracy could be improved by training the models on a more diverse data set — one that Daneshjou and her collaborators created — and by making some modifications to the program.
“When you give an AI program some additional examples of diverse data, and then you test this program on diverse data, it starts doing better,” Daneshjou said. “It’s probably not that different from humans. If you start to train humans properly to be able to recognize skin cancers across diverse skin tones, they would get better at doing so.”